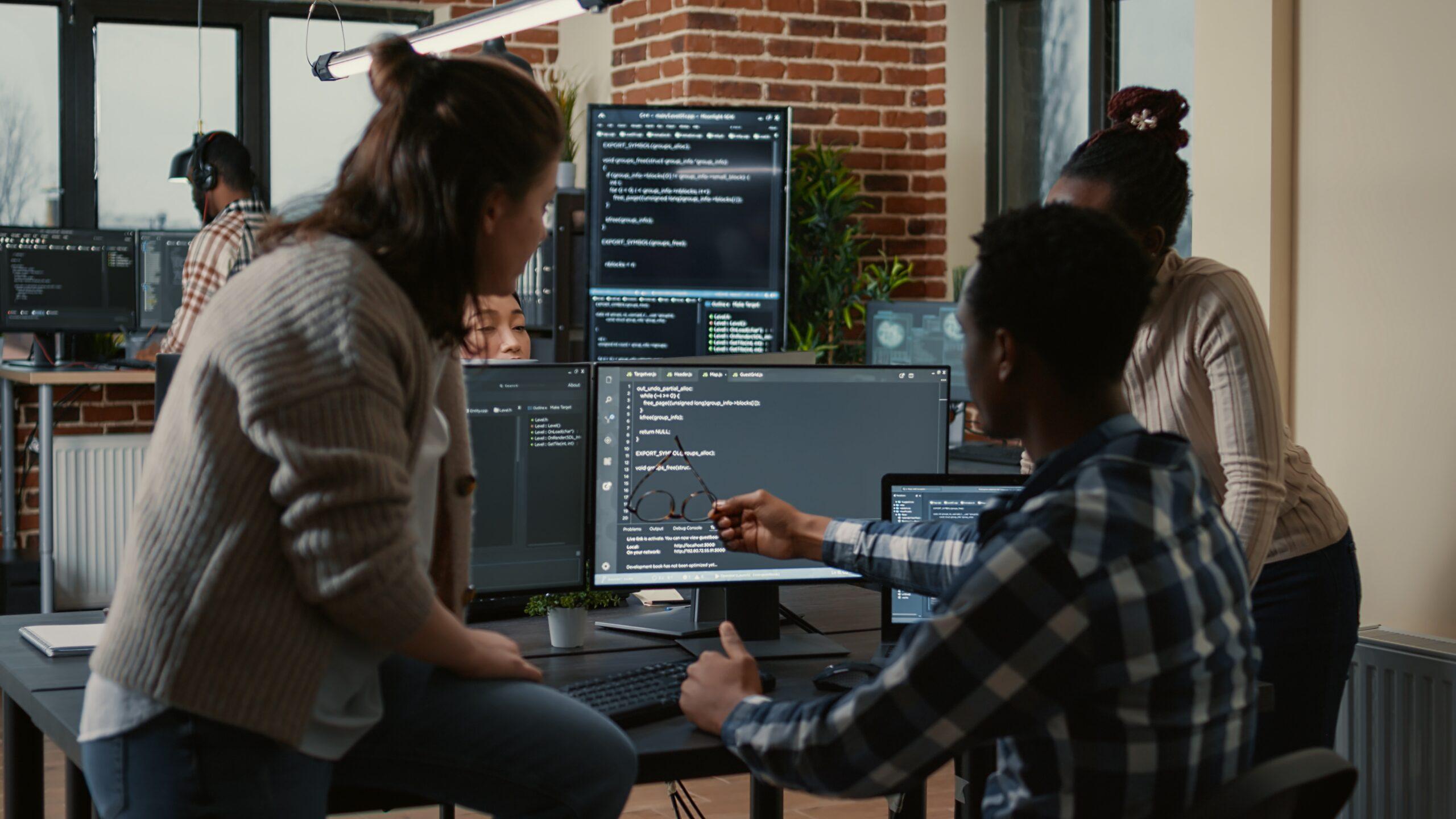
Hiring + recruiting | Blog Post
Data Engineer vs Data Scientist: The Foundation of Data-Driven Decision-Making
Laura Berlinsky-Schine
Share this post
“When you go with your gut or make seemingly random decisions based on instinct, you’re sending an unfortunate message,” writes Allison Arzeno, CEO of Assurance IQ. “You’re telling your team that your whims and interests are more important than making evidence-based, objective decisions.”
In a data-driven world, there is an abundance of information available to us. We should never be relying on our gut to make critical decisions that affect our companies. But businesses can’t use raw, unstructured data alone. Data must be accessible and meaningful. This is even more important in the age of AI. That’s why the need for data-driven professionals is mounting.
Professionals like data engineers and data scientists transform the information into usable insights. Businesses use this data to inform their strategies, overhaul operations, and become more profitable.
Data Engineer vs Data Scientist
At one point, data scientists performed the role of data engineer. The technology simply wasn’t advanced enough to warrant the demand for two separate roles. Even today, in some organizations, particularly smaller ones, these roles continue to overlap.
But increasingly, we’re seeing the responsibilities divided across two separate jobs. The data field is becoming more complex and demands a variety of skilled and specialized professionals, particularly in larger and more complex organizations.
A Big Data Landscape
We live in a big data landscape. It has changed how we collect, evaluate, and utilize information. With so much information available, we are better equipped to glean insights and uncover trends.
Big data extends across industries and businesses. It’s essential for driving efficiency and profits. Now, it is used for everything from education to environment and sustainability.
Consider the UN Global Pulse initiative. This project involved leveraging big data to promote sustainable development. Through the Big Data Climate Challenge, global projects leveraged big data to address the impact of climate change.
Big data is central to the roles of both data engineers and data scientists.
Responsibilities
On the surface, data scientists and engineers have similar roles; both work in the data science field and extract meaningful insights from data. And they often collaborate.
However, their responsibilities differ. Data engineers create the systems for managing and organizing data, while data scientists use that data to make informed predictions and fuel better business decisions.
Here’s a closer look at what each tech professional does.
Data Engineer
Data engineers design and build the infrastructure for data systems—the architecture to process, assess, and manage data—such as complex databases. Essentially, they make it possible for data to be available. They ensure the data is valuable, of high quality, and accessible for analysis.
Data engineers use techniques and technologies like ETL (extract, transform, load), machine learning, and statistics to integrate data from multiple sources into a single system. They also monitor that data.
By preparing raw data for modeling, they serve as the starting point for other data specialists, including data scientists, to do their magic. Their work helps data scientists and other professionals access data more easily.
Data Scientist
Data scientists make sense of the data that has been prepared for them by data engineers. They work closely with business leaders and stakeholders to understand their challenges and needs, clean data, and ensure data reliability.
Using techniques, technologies, and tools—including statistics, programming, predictive modeling, machine learning, and complex analytics—data scientists mine big data sets and uncover patterns.
These professionals glean insights so the business can make data-driven decisions and develop hypotheses and predictions to forecast trends. For example, in lending, they might predict the likelihood of defaulting on loans. They also communicate their findings to technical and non-technical stakeholders.
Skills
Data scientists and data engineers need several overlapping skills, such as statistics, business intelligence, problem-solving, data ethics, and analytical skills. They also need to be able to collaborate with other data and technology professionals.
In addition to these, each professional must have a variety of other skills.
Data Engineer
A data engineer requires exemplary technical skills, including a deep knowledge of coding, building data warehouse architectures, and working with cloud platforms. They should be adept with programming languages such as Python, Java, Scala, and SQL. They should also have experience working with databases, data warehouses, and related technologies, such as MySQL, SAP, Oracle, PostgreSQL, and MongoDB. Some examples of data warehouse platforms they might use include Amazon Redshift and IBM Db2 Warehouse.
Data Scientist
There are several overlapping skills that both data scientists and data engineers should have. For example, data scientists also need to have experience with programming languages, such as Python, Java, Scala, R, SQL, and Julia.
Data scientists also need a strong background in statistics and should know data structures, algorithms, and wrangling. They use data visualization tools like Tableau and must be able to communicate their findings to stakeholders, including those without highly technical skills. This informs leaders’ ability to make critical business decisions.
Additionally, data scientists should have experience with machine learning and deep learning. Industry knowledge can also be helpful.
Career Path and Earning Potential
Data engineers and scientists tend to have comparable earning potential, hovering around mid-$100,000 on average, depending on experience, education, location, and other factors.
Both have the potential for thriving careers and are seeing tremendous demand. Career growth for data scientists is projected to be 36% between 2023 and 2033, much faster than average for all occupations.
Whether you’re considering pursuing a career in data science or want to hire a specialist for your team, it’s important to understand the distinctions. Data engineering is more akin to software engineering than data science. Some data engineers start as software engineers. Ultimately, they may become lead architects and manage teams. Data scientists often start in more junior roles, such as data analysts. They may also grow into management roles.
At the end of the day, both data engineers and data scientists are critical to using data effectively and efficiently and helping you make more informed business decisions.
Looking to hire a data engineer or data scientist? Whether you need freelancers, full-timers, or an entire team, we can help. Hire pre-vetted professionals in Latin America, Canada, or Europe from Terminal’s elite talent pool. Get started by browsing elite professionals for free